
Prediction and classification of respiratory motion / Suk Jin Lee, Yuichi Motai
- 作者: Lee, Suk Jin
- 其他作者:
- 出版: Heidelberg : Springer 2014
- 叢書名: Studies in computational intelligence ;525
- 主題: Radiotherapy, Computer-Assisted--methods , Algorithms. , Reproducibility of Results , Respiratory Mechanics. , Respiratory organs--Mechanical properties--Mathematical models , Respiration--Measurement
- ISBN: 9783642415081 (hbk.) :: US$136.66 、 3642415083 (hbk.)
- 一般註:Includes bibliographical references
-
讀者標籤:
- 系統號: 005206986 | 機讀編目格式
館藏資訊
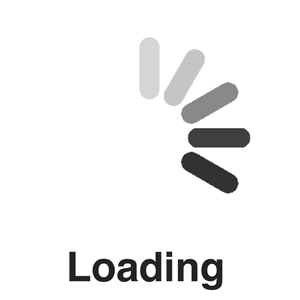
摘要註
This book describes recent radiotherapy technologies including tools for measuring target position during radiotherapy and tracking-based delivery systems. This book presents a customized prediction of respiratory motion with clustering from multiple patient interactions. The proposed method contributes to the improvement of patient treatments by considering breathing pattern for the accurate dose calculation in radiotherapy systems. Real-time tumor-tracking, where the prediction of irregularities becomes relevant, has yet to be clinically established. The statistical quantitative modeling for irregular breathing classification, in which commercial respiration traces are retrospectively categorized into several classes based on breathing pattern are discussed as well. The proposed statistical classification may provide clinical advantages to adjust the dose rate before and during the external beam radiotherapy for minimizing the safety margin. In the first chapter following the Introduction to this book, we review three prediction approaches of respiratory motion: model-based methods, model-free heuristic learning algorithms, and hybrid methods. In the following chapter, we present a phantom study/prediction of human motion with distributed body sensors using a Polhemus Liberty AC magnetic tracker. Next we describe respiratory motion estimation with hybrid implementation of extended Kalman filter. The given method assigns the recurrent neural network the role of the predictor and the extended Kalman filter the role of the corrector. After that, we present customized prediction of respiratory motion with clustering from multiple patient interactions. For the customized prediction, we construct the clustering based on breathing patterns of multiple patients using the feature selection metrics that are composed of a variety of breathing features. We have evaluated the new algorithm by comparing the prediction overshoot and the tracking estimation value. The experime